Calibration of fuel performance codes – treating model inadequacies, nuisance parameters, and unrecognized systematic uncertainties
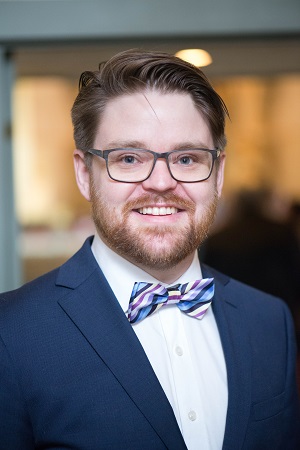
Research host
Uppsala University, Department of Physics and Astronomy, Applied Nuclear Physics, Nuclear reaction group, and Fission diagnostics group
Research done by doctoral student
Gustav Robertson
Main supervisor
Henrik Sjöstrand
Co-supervisor
Peter Andersson and Paul Blair
Formal project start
2020-09-01
Expected time of completion
2024-09-01
Discipline
Fuel performance modeling, statistics
Keywords
Nuclear Fuel, Cladding Oxidation, Machine Learning, Calibration, Inverse-UQ, Data Assimilation
Motivation
The proposed project addresses challenges in the calibration of fuel performance codes. These codes include models that predict the thermo-mechanical behavior of the fuel and hence, the performance and safety functions of the fuel for regular reactor operation, anticipated operational occurrences, accidents, and back-end applications.
There are several challenges with acquiring calibrated predictive models with well-founded uncertainty estimates. These challenges include handling interlinked models; integral, biased, and sparse calibration data; various types of input uncertainties; computationally costly executions; and model inadequacies. Therefore, inverse uncertainty quantification (UQ) in fuel performance modeling is particularly challenging.
Specifically, the UQ within fuel performance simulations is crucial in establishing plant operation safety limits. This manifests as conservative estimates of operation limits or an evaluation showing that the fuel cladding barrier will not be breached for a given plant operation. In addition, the fuel rod behavior plays a central role in accident analyses, for example, in evaluating loss-of-coolant accidents where the cladding embrittlement is a direct safety-related parameter. In this context, calibration plays a pivotal role as it defines the uncertainties when providing the conservative estimate. Inaccuracy in calibration can lead to overstepping established failure limits, which is not acceptable from a safety standpoint. Conversely, an overly cautious and conservative treatment causes less efficient operation and fuel utilization with cost and increased waste disposal impacts.
One of the most demanding challenges in model calibration is caused by so-called model inadequacies. A model inadequacy is when the model cannot recreate the physical reality independent of the choice of model parameters. This often has severe consequences if not accounted for properly. A simple example is shown in Figure 1, where a linear model (blue line) is used to estimate a more complex reality (orange, broken line). It is clearly seen that the resulting blue uncertainty bands of the model do not reflect the error of the model. This is the result that would be obtained if the model inadequacy is not accounted for, with significant safety implications if the model was to predict a nuclear engineering safety parameter.
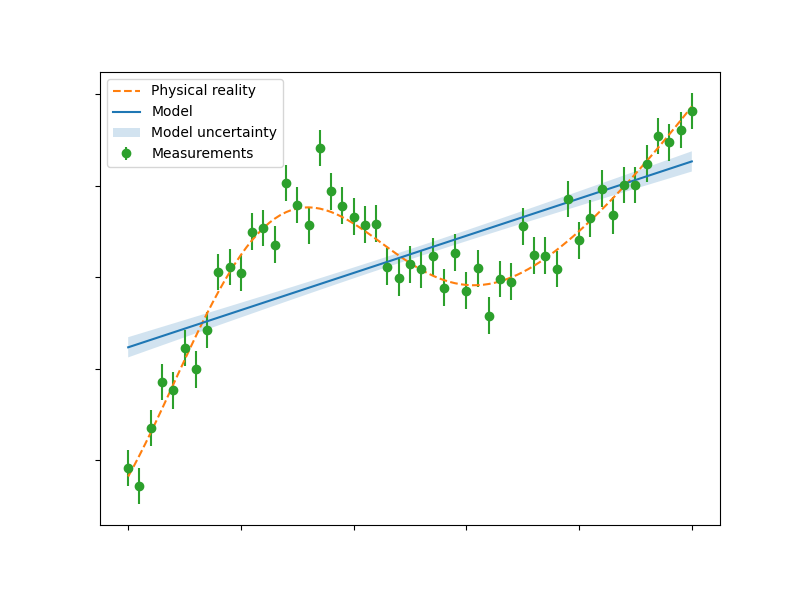
Fuel performance codes determine cladding oxidation, hydrogen pickup, and gas release, among other quantities. These phenomena, if not limited, can have a direct negative impact on fuel safety. For example, hydrogen uptake harms the mechanical properties of the zirconium alloy, cladding oxidation consumes the cladding, and the oxide is a much poorer heat conductor. In addition, the modeling of fission gas release is important since fission gases degrade the thermal conductivity of the gas inside the fuel rods and increase the internal pressure. Higher temperatures may also lead to negative thermal feedback since a depressed thermal conductivity will, in turn, enhance additional fission gas release. These three quantities have been the primary focus of the studies performed within the project so far.
Moreover, the project develops competence in machine learning and computational methods. This has an added advantage in that the project can cross-fertilize neighboring nuclear technology projects in the sphere of SKC.
To summarize, the project aims to ensure safety via reliable quantification of margins simultaneously as it enables efficient use of fuel and benefits all partners of SKC. This will be achieved by improving techniques for calibration and UQ in the context of fuel rod performance simulations.
Key findings this far
In our first paper, we present a synthetical study to demonstrate the adverse effect of model inadequacy when calibrating cladding oxidation and hydrogen pickup models. We discuss schematically different approaches to address unknown uncertainties in calibration. We point out that the most integrable way in the context of existing licensing methods is to assign their uncertainties to the model parameters. For that purpose, we present a Bayesian technique based on Markov Chain Monte Carlo (MCMC) that adapts the model parameters' covariance to explain the distribution of the residuals.Compared to the schematical figure above, the counterpart of uncertainty inflation is depicted in Figure 2.
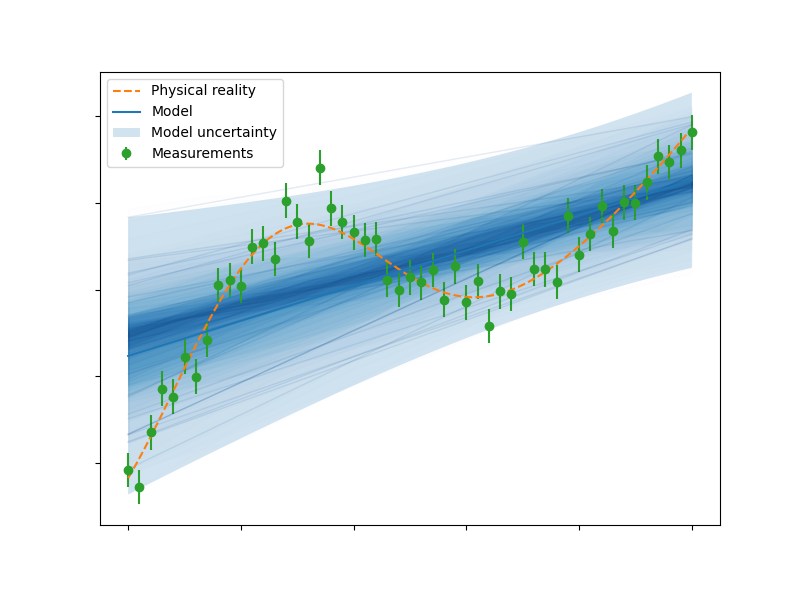
The method is repeatedly validated using different synthetic calibration and validation data sets, including a controlled amount of model inadequacies. The overall conclusion is that the presented method is a good candidate for addressing model inadequacies in the calibration of fuel performance models.
MCMC techniques take many samples to converge and often require a surrogate model. In our first work, we see that model inadequacy treatment depends on both affordable evaluations of the code and the corresponding Jacobian. Therefore, in our second application, we investigate the use of differentiated Gaussian Processes (GPs) as surrogates to be used in place of a fission gas release model. We see that the GPs adapt well to the fission gas release model with a low validation error on average. The benefit of using differentiated GPs is twofold; it offers cheap predictions of the code's output and the corresponding derivatives.
Publications from this project
Publications
Robertson, G., Sjöstrand, H., Andersson, P., Hansson, J., & Blair, P. (2022). Treating model inadequacy in fuel performance model calibration by parameter uncertainty inflation. Annals of Nuclear Energy, 179, 109363. https://doi.org/10.1016/j.anucene.2022.109363
Robertson, G., Sjöstrand, H., & Andersson, P. (2022). Surrogate Modeling with Derivative Prediction for Implementation in Inverse Uncertainty Quantification Methods for Fuel Performance Modeling. TopFuel 2022 Light Water Reactor Fuel Performance Conference.
Presentations
Robertson, G. (2020). CaNel – Calibration of Nuclear fuel performance codes: Machine learning for nuclear safety. KTH Energy Dialogue 2020, 19 November, online. http://urn.kb.se/resolve?urn=urn:nbn:se:uu:diva-434785
Robertson, G. (2020). CaNel – Calibration of Nuclear fuel performance codes: Treating model inadequacies, nuisance parameters and unrecognized systematic uncertainties. SKC Symposium 2020, 20-21 October, online. http://urn.kb.se/resolve?urn=urn:nbn:se:uu:diva-434784
Robertson, G. (2021). CaNel—Calibration of Nuclear fuel performance codes: 6-month seminar. Seminar at Westinghouse Electric Sweden AB. http://urn.kb.se/resolve?urn=urn:nbn:se:uu:diva-453582
Robertson, G. (2021). CaNel—Calibration of Nuclear fuel performance codes: Addressing model inadequacy in fuel performance modelling. TopFuel 2021. http://urn.kb.se/resolve?urn=urn:nbn:se:uu:diva-467969
Robertson, G. (2021). CaNel—Calibration of Nuclear fuel performance codes: Treatment of model inadequacies when calibrating cladding oxidation and hydrogen pickup. Transuranus User's Group Meeting, 2021. http://urn.kb.se/resolve?urn=urn:nbn:se:uu:diva-453556
Robertson, G. (2021). CaNel—Calibration of Nuclear fuel performance codes: Accounting for model inadequacy using a hierarchical Bayesian approach. SKC Symposium. http://urn.kb.se/resolve?urn=urn:nbn:se:uu:diva-468023
Robertson, G. (2021). CaNel—Calibration of Nuclear fuel performance codes: Accounting for model inadequacy using a hierarchical Bayesian approach. SKC Symposium. http://urn.kb.se/resolve?urn=urn:nbn:se:uu:diva-468023
Robertson, G. (2021). The golden combination of MCMC and Gaussian processes for calibration. SAINT - Swedish academic initiative for radiation sciences and nuclear technology. http://urn.kb.se/resolve?urn=urn:nbn:se:uu:diva-434783
Robertson, G., Sjöstrand, H., Andersson, P., & Blair, P. (2021). CaNel—Calibration of Nuclear fuel performance codes: A hierarchical Bayesian approach to account for model inadequacy. Nuclear Futures Bangor, NuFuel 2021, Bangor University, 3th-16th September, 2021, Bangor. http://urn.kb.se/resolve?urn=urn:nbn:se:uu:diva-453547
Robertson, G. (2022). CaNel—Calibration of Nuclear fuel performance codes: Addressing Model Inadequacy in Inverse Uncertainty Quantification for Fuel Performance Modeling by using a Hierarchical Statistical Formulation. SIAM UQ22. DiVA. http://urn.kb.se/resolve?urn=urn:nbn:se:uu:diva-484172
Robertson, G. (2022). Surrogates with gradient prediction for inverse uncertainty quantification in fuel performance modeling. SAINT 2022. DiVA. http://urn.kb.se/resolve?urn=urn:nbn:se:uu:diva-484173
Collaboration partners
Westinghouse Electric Sweden AB. Project partner. Supports the project by letting the project use codes, computational resources, and calibrate/validate against real-world measurement data.
Paul Van Uffelen, DG Joint Research Centre-JRC Directorate G – Nuclear Safety & Security Unit G.I.5 Nuclear Science and Applications. JRC has provided the fuel performance code Transuranus and supports with technical consultation regarding various models.